AI-supported quality assessment of hazelnuts and dried fruit
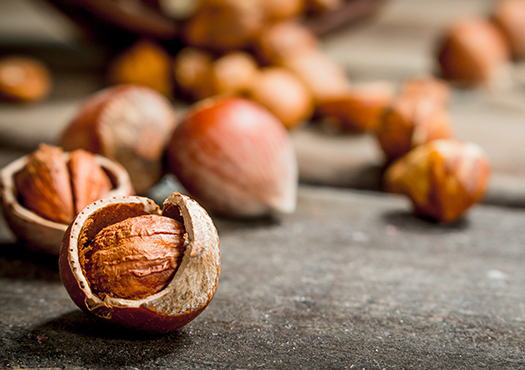
Minimizing the health risk by reducing mycotoxin contamination
Agricultural raw materials are subject to a variety of influences that have a significant impact on product quality and can also affect consumer safety. In particular, nuts or products dried in the countries of origin, such as dried fruit, have an increased risk of mold infestation due to longer storage periods. In order to reduce consumer exposure to the carcinogenic mycotoxins formed by molds and to minimize the associated health risk, various sorting methods are used in food production and processing. Depending on the method used and the product being sorted, this application is cost-intensive or does not achieve the required sensitivity to reliably separate contaminated goods.
AI-supported and non-destructive quality assessment of hazelnuts using gas sensors
As part of the transnational joint project RAVOC, we are working with a Turkish research partner to develop a classification method for hazelnuts based on gas sensor technology, which should enable non-destructive differentiation between nuts contaminated with mold and high-quality nuts. By using machine learning methods, the model developed at the Fraunhofer IVV will be highly robust in relation to naturally occurring fluctuations in the product and microflora.
For this purpose, the Fraunhofer IVV uses gas chromatography to determine all volatile substances (volatolome) emitted from hazelnuts from different regions of Turkey with different microbial contamination and supplements them with other reference parameters determined in the laboratory (e.g. mold contamination and mycotoxin content). At the same time, hazelnuts, which exhibit increased fatty acid degradation, are also included in the analyses in order to obtain a high specificity of the model for mold contamination. The integration of measurement data from analytical infrared spectroscopy into the AI-supported model to improve performance is also planned in this project.
The collaborative research project led by the Fraunhofer IVV combines the expertise of two internationally relevant countries for hazelnut cultivation and processing and also directly incorporates the needs of future users in its development.
Transfer of the classification model for risk assessment to other raw materials
The technical solutions currently available on the market for classifying and sorting raw materials often have a decisive disadvantage due to their focus on one sample type, such as hazelnuts. The methodology is not easily transferable to other products. This CORNET project therefore aims to investigate the possibility of transferring the classification model generated from the volatolomics data of hazelnut samples to other raw materials such as dried fruit. For this purpose, the emitted volatile substances and other data will also be collected from dried figs with different microbial loads and the validity of the previously established model will be determined for this sample matrix.
Based on the results of this joint project, the development of simple AI-supported gas sensor arrays is to be made possible, which are particularly suitable for the non-destructive sampling of various raw materials in large-volume containers (e.g. big packs) in incoming goods.
Project term: |
2024 to 2026 |
Project management / financing: |
Project sponsor DLR (via Industrial Association for Food Technology and Packaging IVLV), Federal Ministry of Economics and Climate Protection, Cornet / IGF international |
Official title: |
Rapid Quality Assessment of Nuts and Dried Fruits by Volatolomics |
Funding code: |
01IF00401C |